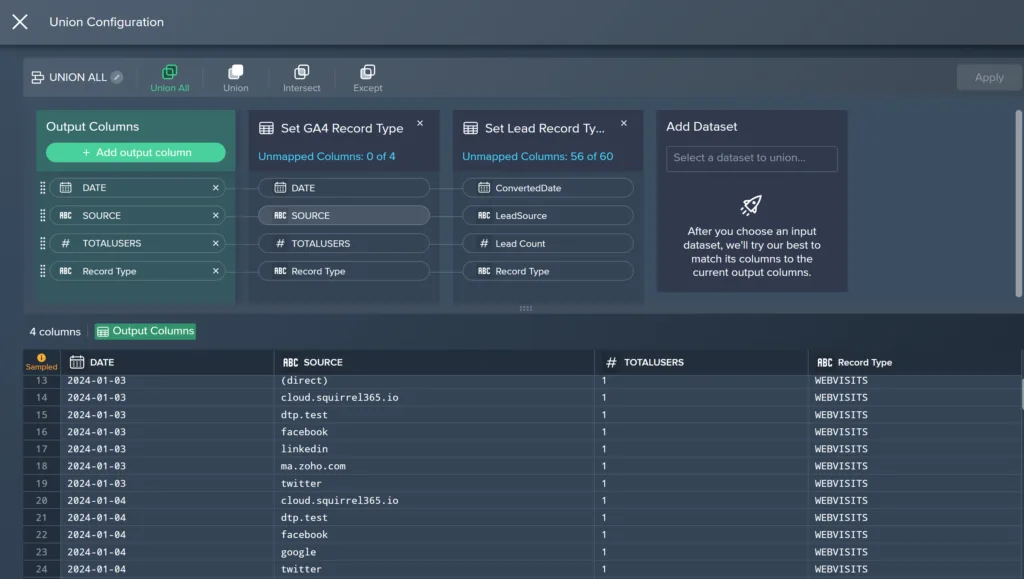
For this month’s DataTools spotlight, I wanted to share my long time favorite for Snowflake tool, Datameer. Years ago, I found Datameer solved my slow Snowflake adoption problem. My team was loaded with requirements but had only one data engineer on staff. Historically we used Alteryx and Tableau prep to get by. Extracting data from Snowflake to transform and insert into Snowflake was a pattern that didn’t make sense. Years ago, I shared the story how slow adoption turned around with Datameer where we delivered 20 models to production in a couple of months. That resulted in us turning off Tableau Prep and Alteryx.
As that story progressed, times got tough and we had to do more with fewer human resources. Datameer was the only way we could keep up with change management and new requests. Those are the stories you don’t lead with as case study, but I can openly share that experience now with my own clients as DataTools Pro.
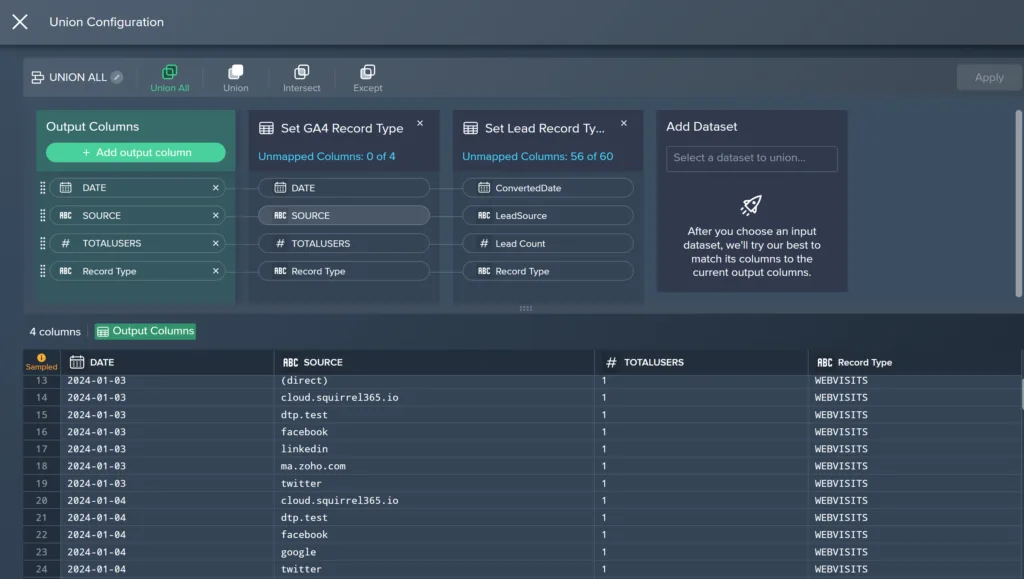
In 2024, I continued writing about my Snowflake experiences with Datameer. In the last year, Datameer has continued to level up its enterprise grade features while continuing to add time savings features for individual contributors.
When I use Datameer for Snowflake
There are three primary scenarios where Datameer makes sense for Snowflake customers.
- Accelerating Snowflake adoption and deployment of data models
- Empower analysts on Snowflake while keeping data in Snowflake
- Eliminate self-service data prep tech debt
To elaborate on this third point, I have recently run into massive, complex Tableau + Alteryx self service installations. I love Alteryx and Tableau for data exploration and dashboards as an individual contributor. However, watching enterprises lose control over the volume, velocity, and cost of self-service is painful to see.
In recent years, the pendulum has swung back, as companies have invested in modern data platforms like Databricks, Snowflake, Fabric, and Google Cloud Platform. When it comes to Snowflake, as a practitioner, I haven’t found anything that makes it easier than Datameer to wrangle and prepare data in Snowflake for business consumption. I have started chronicling how to convert Alteryx to SQL Snowflake.
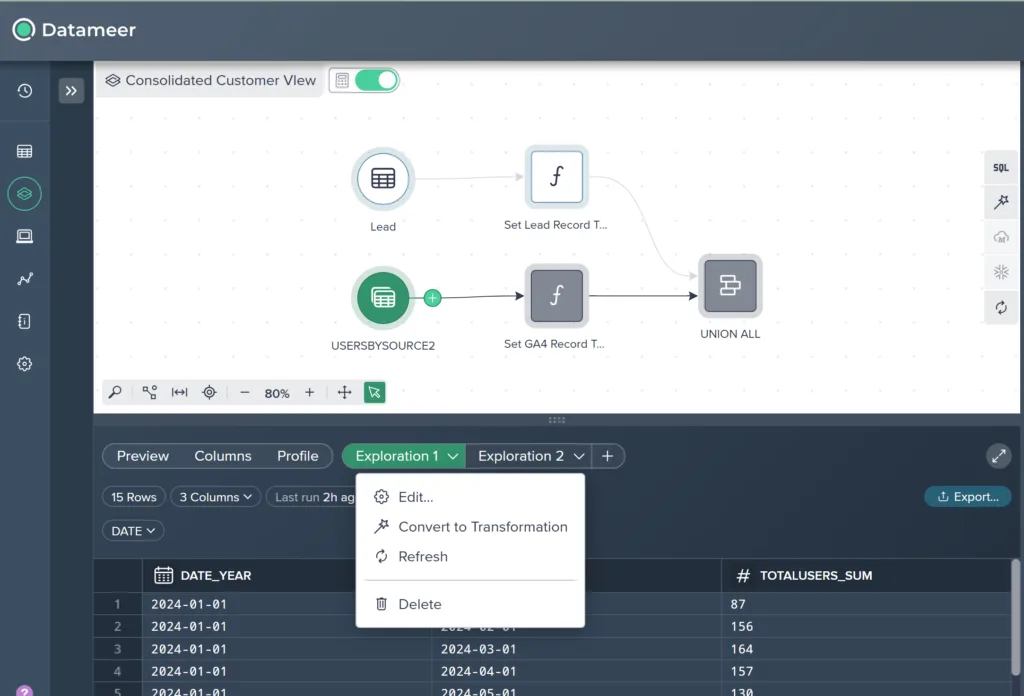
Enterprise Snowflake tool features that matter
From scheduled data delivery via email to publishing fact tables for business intelligence tools like Tableau and Power BI, Datameer provides speed, control, governance, lifecycle management, and cost management in one package.
- Native Snowflake means data remains in Snowflake
- A user experience built for speed
- No-code where you want it, low-code where you need it!
- Seamless SDLC, code rollback and environment promotion management
- Generate views or materialize tables
- Built in data validation and exploration
- Removes proprietary tool lock-in and points of failure.
- Predictable enterprise pricing
I was happy to be an early adopter of Datameer while working for a small enterprise. Now, I get to use Datameer once again with medium and large enterprises. If you are on Snowflake or making the move to Snowflake and need to accelerate adoption, feel free to reach out, and I’d be happy to give you a walkthrough.
Previous Spotlight
DataTools Pro Spotlight: UTM Grabber
UTM Grabber is used by 100,000 websites to handle organic and paid web tracking, boasting over 30 attributes that can be passed from your WordPress forms into your CRM like Salesforce. We use and recommend UTM Grabber for any company using WordPress. Why use UTM Grabber? When you have a web to lead form, there…